Entry Descent and Landing (EDL) technologies used for robotic landings on Mars have been derived and extended from the capabilities developed for Apollo during the 1960s and 1970s. However, landing humans on Mars (or Europa for example) is going to pose unprecedented challenges to EDL technologies driven by the need to land a very large payload mass with extremely high reliability and safety requirements.
In order to design the next generation of EDL systems, it is necessary to analyze a wide range of architectures with high fidelity simulations of performance, cost, schedule and risk that take into account uncertainty. Currently, performance analysis of EDL systems under uncertainty
is done using Monte Carlo techniques. The large datasets generated are manually analyzed by the subject matter expert, trying to find interesting correlations and couplings between parameters, and assess the sensitivity of figures of merit to various parameters. This analysis work is important
since it may lead to the discovery of a major flaw in a design, for example.
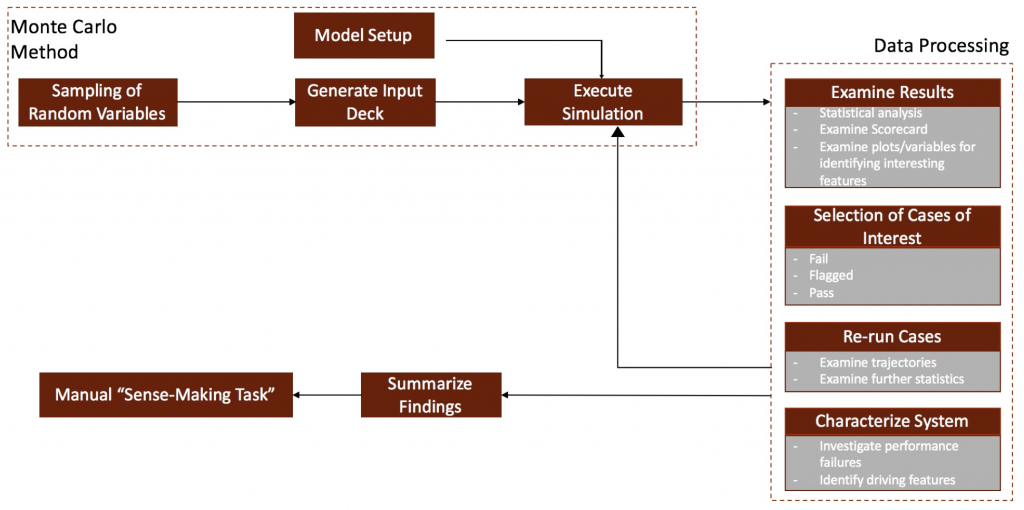
However, the current approach suffers from an important limitation. While the subject matter expert can leverage his or her knowledge and expertise with past systems to identify issues and features of interest in the dataset, the next generation of EDL systems may present unprecedented challenges that may be missed by the human.
On the other hand, while there have been great advances in statistical machine learning and sensitivity analysis that enable increased automation (see for example the automatic statistician), reliable and fully automated mining of the dataset for relevant and interesting knowledge is still unfeasible, especially for unprecedented systems. Therefore, this project explores mixed-initiative approaches for intelligent data understanding of EDL simulations.
To enable the mixed-initiative approach, we are developing a cognitive assistant largely based on Daphne. The architecture of the Daphne cognitive assistant showing the EDL-related modules can be seen below.
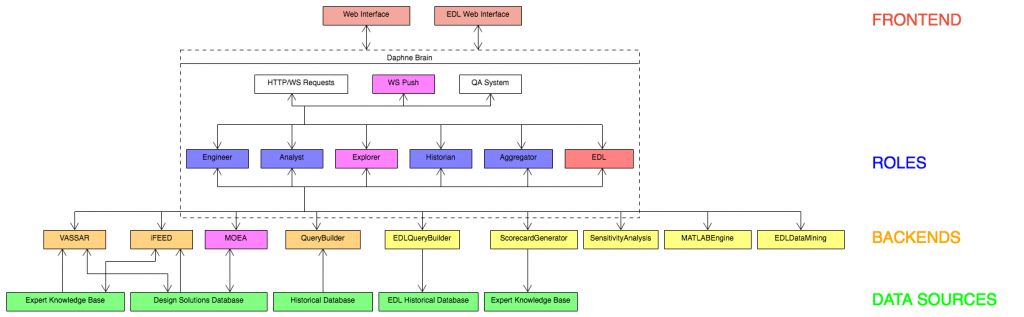
In addition to loading and visualizing simulation result files and generating scorecards to see a summary of requirements that are either not satisfied or on the verge of not being satisfied, the Daphne/EDL skill has rule extraction and sensitivity analysis algorithms that facilitate the process of figuring out why a particular requirement is not being satisfied. It also has access to a database of past EDL systems, so that one can ask questions about various missions parameters and compare to the ones from the simulations at hand.
Learn more about this project in the publications below.
Santini De León, S., Selva, D., & Way, D. W. (2019). A Cognitive Assistant for Entry, Descent, and Landing Architecture Analysis. IEEE Aerospace Conference Proceedings.
This project is funded by the NASA NSTRF program.